Abstract:
In this study, a hybrid tweet classification system built using Word2Vec and Support Vector Machines is presented. The aim of the study is to use the tweet classification system to solve the natural language processing task of automatically assigning labels to unseen tweets into three label attributes namely, anti-zuma, neutral and pro-zuma. Model building process was made up of 3 different phases, namely human tweet labeling, tweet encoding and SVM-based model training. Human tweet labeling involved the selection of 3100 tweets and allocate them to two human coders for labeling into one of three attributes named above. Tweet encoding involved the using the Word2Vec algorithm to train a word embedding based on all the tweets that constituted our dataset. The resulting high-dimensional word vector representations was then used to encode the labeled tweets before using the vectors for training the SVM-based tweet classifier. As a final step, we trained an SVM-based tweet classification system and used the model to predict classes for all unseen tweets that made the bulky of our dataset. Our hybrid tweet classification model achieves an overall F1-score of 77% which is state-of-the-art considering the diversity and complex nature of language commonly used in tweets. Qualitative analysis demonstrated the ability of the model to correctly classify tweets written in Japanese.
Keywords: Word2Vec, Support Vector Machines, tweet encoding, tweet classification system
My first paper with Stellenbosch University affiliation was published in Quantum Science and Technology.
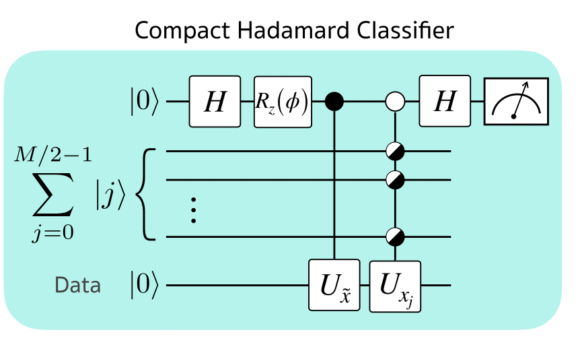
Abstract: Quantum computing opens exciting opportunities for kernel-based machine learning methods, which have broad applications in data analysis. Recent works show that quantum computers can efficiently construct a model of a classifier by engineering the quantum interference effect to carry out the kernel evaluation in parallel. For practical applications of these quantum machine learning methods, an important issue is to minimize the size of quantum circuits. We present the simplest quantum circuit for constructing a kernel-based binary classifier. This is achieved by generalizing the interference circuit to encode data labels in the relative phases of the quantum state and by introducing compact amplitude encoding, which encodes two training data vectors into one quantum register. When compared to the simplest known quantum binary classifier, the number of qubits is reduced by two and the number of steps is reduced linearly with respect to the number of training data. The two-qubit measurement with post-selection required in the previous method is simplified to single-qubit measurement. Furthermore, the final quantum state has a smaller amount of entanglement than that of the previous method, which advocates the cost-effectiveness of our method. Our design also provides a straightforward way to handle an imbalanced data set, which is often encountered in many machine learning problems.
The latest paper with Vinayak Jagadish and R. Srikanth was just published in Physical Review A.
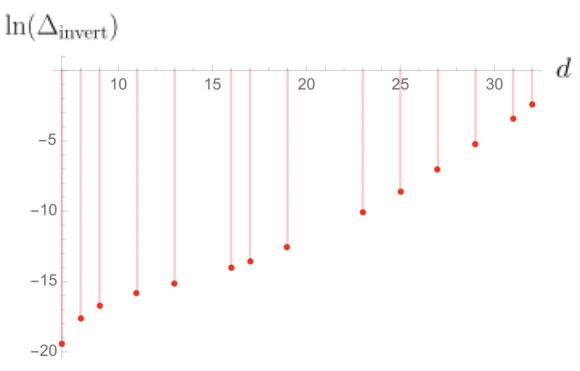
Abstract:
Quantum-access security, where an attacker is granted superposition access to secret keyed functionalities, is a fundamental security model and its study has inspired results in post-quantum security. We revisit, and fill a gap in, the quantum-access security analysis of the Lamport one-time signature scheme (OTS) in the quantum random oracle model (QROM) by Alagic et al. (Eurocrypt 2020). We then go on to generalize the technique to the Winternitz OTS. Along the way, we develop a tool for the analysis of hash chains in the QROM based on the superposition oracle technique by Zhandry (Crypto 2019) which might be of independent interest.
Prof Francesco Petruccione joined Stellenbosch University on 1 May 2022.
Welcome to quantum.sun.ac.za. The page will display all things “quantum” at Stellenbosch University.